Modeling the effect of HIV/AIDS stigma on HIV infection dynamics in Kenya.
This project is in collaboration with the Masamu Advanced Study Institute (MASI). MASI is an international institute funded by the National Science Foundation with the goal of fostering mathematical collaboration between researchers from the United States and those in Southern Africa.
Background
HIV/AIDS-related stigma and discrimination continues to impede the progress of HIV/AIDS response across the world. People living with HIV/AIDS (PLWHA) who experience high levels of stigma avoid testing and delay initiating care and treatment.
Many researchers have formulated mathematical models to understand the dynamics of HIV/AIDS, but few have looked at the social issues affecting the dynamics of HIV/AIDS like stigma and discrimination. We construct an infectious disease model for HIV/AIDS to investigate the effects of stigma as it pertains to treatment rates, death rates, and long-term disease projections in Kenya.
To model the disease we use a compartmental structure in which the total population is divided into compartments which reflect the HIV/stigma status of individuals at time t. Individuals are classified into the following categories, using the number of individuals in each compartment: susceptible to HIV, infected with HIV but not being treated (with or without stigma), and infected and being treated for HIV. We also include the fraction of newly infected who exhibit stigma-related behaviors as a state variable σ(t) that changes over time as well as a scenarios in which σ is a positive constant. The model structure was chosen to help us address the model questions posed above and can be modified to apply to other countries or stigmatized diseases.
With these assumptions we obtain the model in System 1, which is based on the schematic presented in Figure 1.

Data Collection and Use
Select studies have measured stigma through interviews with PLWHA asking how many times or how often they have experienced various forms of discrimination over the past year. We obtained detailed results from three separate Demographic and Health Surveys conducted by Kenya’s National Bureau of Statistics for the years of 2003, 2008, and 2013. Each of surveys include repeated questions related to individual perceptions of HIV/AIDS that were used to measure the rate of change of stigma in Kenya over time.
We also obtained time series data for HIV/AIDS in Kenya from 1990-2019. The data includes total number of cases, deaths due to HIV/AIDS, and percent of individuals with the disease that are seeking treatment. While some of the parameters in our model were obtained from the literature, others were estimated by fitting our model to this case data. Specifically, we formulate an optimization problem that minimizes the difference between model output and the data. We implement the optimization problem in matlab using the built-in fmincon algorithm, which allows us to determine the set of parameters that best match the data.

Specific Outcomes
Our project is unique in that we are considering how the social issue of stigma affects the dynamics of HIV/AIDS in Kenya. We carried out the follow three analyses in our peer reviewed publication.
We first calculated all equilibrium points in the model. Equilibrium points, or fixed points, are solutions to the differential equations that do not change over time. Equilibrium points of particular interest include the disease-free equilibrium, which provides solutions to the model in which the disease will eventually dissipate from the system, and the endemic equilibrium, which provides solutions where the disease will persist indefinitely. Analyzing the stability of these points is particularly crucial to understanding dynamics and future projections.
We then used the equilibrium points to calculate the key value known as the Basic Reproduction Number. The value can essentially be thought of as the expected number of subsequent new cases directly produced by each infected individual. One can think of as boiling down a complicated disease model to a single number. This value is extremely important and useful in any infectious disease model as it can be used to determine conditions that will cause the disease to spread or go away.
Finally, we used the parameters we estimate from the data we have collected to run simulations that consider different potential scenarios. For instance, we considered a scenario where stigma changes over time using the function we fit to survey data, a scenario where stigma is constant over time, and a scenario where there is no HIV/AIDS-related stigma. See the figure below for example outcomes and see our publication in Mathematics for more details.

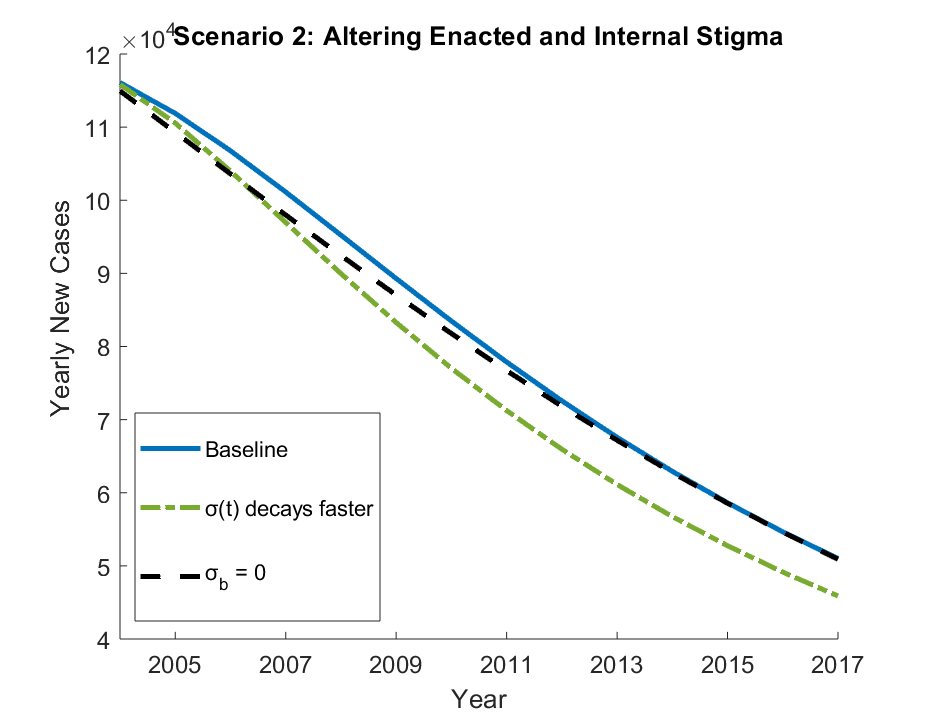
留言